by Jerry Cole
Linked paper: Automated bird sound classifications of long-duration recordings produce occupancy model outputs similar to manually annotated data by Jerry S. Cole, Nicole L. Michel, Shane A. Emerson, and Rodney B. Siegel. Ornithological Applications.
New technology is exciting! It’s always fun to brainstorm ways you can use the latest and greatest thing in your research. Autonomous Recording Units, or ARUs, are a great example. As these devices have become more affordable, more ornithologists are considering employing them. It’s hard not to get excited about using tiny computer ears to record bird sounds over long stretches of time, in multiple locations at once, without having to manage the cost and logistics of getting trained human ears out in the field. (While most of us enjoy being outdoors doing point counts, they’re not always affordable or practical to do on a long-term scale.)
The less fun aspect of new technology is the nitty-gritty work of evaluating the quality of the data collected by your new technology, and figuring out how to manage and use that data. For instance, ARUs can record hundreds of hours of sounds with little effort on your part. But it is possible to have too much of a good thing, because someone has to listen to and annotate all those recordings. Now, instead of spending time out in the field listening to birds, you are spending even more time at your desk listening to recordings of birds. Suddenly the ARUs are not so exciting.
This is where another bit of new technology, an “automated classifier” such as BirdNET, can assist. BirdNET, is a freely available comprehensive classifier produced by the Cornell Lab of Ornithology and the Chemnitz University of Technology that uses a convolutional neural network algorithm to rapidly identify a large suite of bird species. Essentially, artificial intelligence (AI) listens to all those hours of recordings, and processes them faster than the human brain can.
But how good is BirdNET at identifying bird sounds on ARU recordings? And how can you use the detections it makes, and their associated confidence scores, in your statistical analyses? In our study published this week in Ornithological Applications, we discuss how to optimize BirdNET’s classification performance and demonstrate how to use its output in a real-world application.
Our application was a bird monitoring project done in collaboration with the National Audubon Society and California State Parks in the Carnegie State Vehicular Recreation Area, a state park in northwestern California. This project employed ARUs and occupancy modeling—a statistical method that estimates how likely a bird or other organism is to use a particular place. This type of modeling is widely used to determine bird-habitat associations, population trends, bird distribution and conservation status. Occupancy modeling allows us to account for imperfect detection of a bird—for example, when a bird is present, but not vocalizing when an observer visits—however, this requires repeated visits to a sampling site to estimate detection probability. ARUs are particularly suited to estimating detection probability because they stay onsite and can be programmed to record at certain hours every day, or any desired interval.
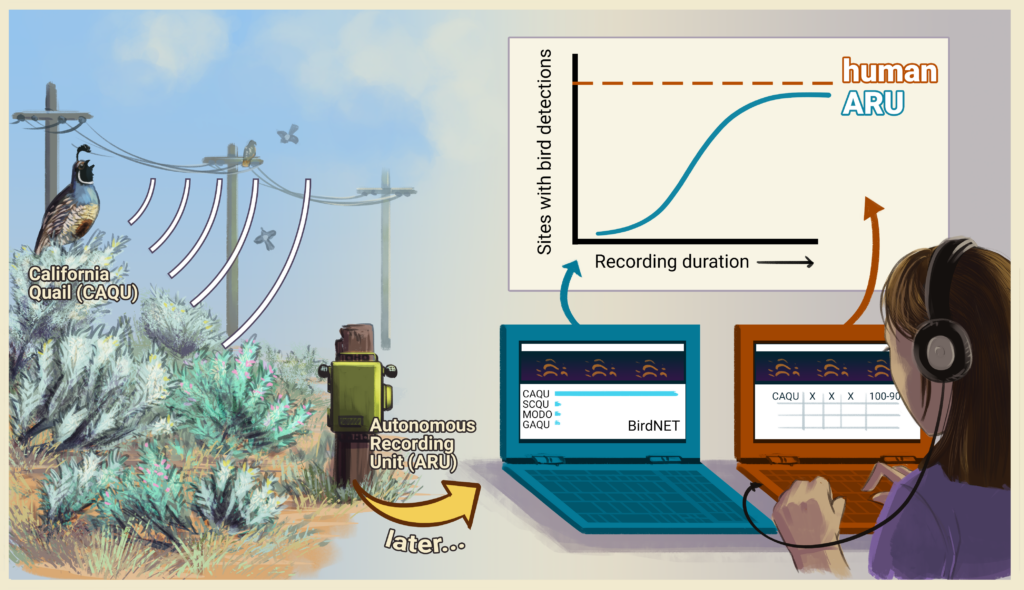
We compared birds detected by a human listener and those detected by BirdNET on the same ARU short duration recordings to evaluate BirdNET’s performance identifying the species found in the study area with its associated environmental noise (wind, traffic noise, etc.) We then used that information to decide how to filter BirdNET’s detections based on their associated confidence scores and discuss how filtering thresholds might vary depending on a project’s goals. In addition, we used BirdNET to annotate long duration recordings and determine the optimal sampling duration to detect the focal species in our study. Lastly we compare occupancy model outputs generated using human-annotated short duration recordings, BirdNET-annotated short duration recordings, BirdNET-annotated long duration recordings and BirdNET-annotated long duration recordings filtered by detection confidence score.
We found that BirdNET detections generated similar occupancy model outputs to human detections and that models which used detections from BirdNET-annotated long duration recordings were the most similar. Filtering detections by BirdNET-generated confidence scores reduced the performance of the models because filtering based on these confidence scores can exclude useful data, thus requiring more/longer recordings to be processed in order to determine occupancy. A limited effort to manually validate BirdNET detections, as we did in this study, allows you to model and account for false positive errors and use unfiltered BirdNET detections.
We conclude that there is good reason to be excited about affordable ARUs and automated classifiers like BirdNET. These tools provide ornithologists with a remarkable opportunity to expand bird research and monitoring efforts, especially in poorly surveyed areas. The data they provide will increase the accuracy and precision of our occupancy, abundance, and population trend estimates. The Institute for Bird Populations (IBP) is currently using ARUs and BirdNET in two additional locations. One project is in a remote field location, and the other requires minimal disturbance of a sensitive bird species, conditions which made ARUs preferable to surveys by human observers. Our hope is that this study will help more ornithologists use these tools effectively to improve our understanding of bird population status and dynamics, and our ability to conserve vulnerable species.
This post was updated on 4/25/22 to correct an omission of the names of partner agencies in the project.
Very timely and useful.