By Ryan C. Burner
Related paper: Informative priors can account for location uncertainty in stop-level analyses of the North American Breeding Bird Survey (BBS), allowing fine-scale ecological analyses by Ryan C. Burner, Alan Kirschbaum, Jeffrey A. Hostetler, David J. Ziolkowski Jr., Nicholas M. Anich, Daniel Turek, Eli D. Striegel, Neal D. Niemuth, Ornithological Applications
Location uncertainty in the North American Breeding Bird Survey (BBS)
The Breeding Bird Survey (BBS) is a long-term monitoring program that collects data on bird populations across North America. Established in 1966, it involves trained volunteers conducting 50 standardized bird counts (‘stops’) along each of thousands of designated routes during the breeding season. The BBS is a vital resource for understanding bird populations and trends across North America. However, one significant challenge that researchers face when using the rich BBS data for finer-scale analyses is location uncertainty.
BBS routes are typically established along roads, and the locations of individual stops along these route paths can vary year to year and route to route due to differences in observers and conditions. Furthermore, precise annual count locations are not reported to the BBS office. This location variability and uncertainty aren’t a problem for estimating large-scale population trends, but this extensive dataset also has much potential for improving our understanding of relationships between birds and land cover at much finer spatial scales. But, location uncertainty makes it difficult to associate bird records from a given point count with specific habitats or land covers.
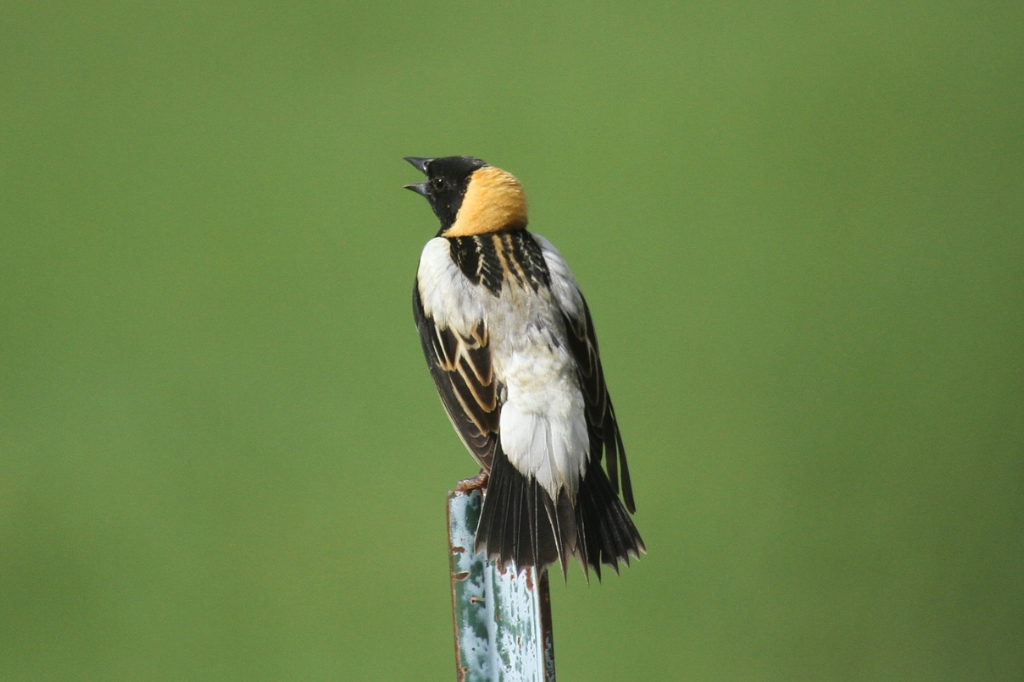
How much uncertainty and how to deal with it?
Our method is based on two insights: First, although locations of stops along a BBS route are not precisely known, they are likely to follow predictable patterns. Second, methods exist for incorporating partial (or ‘probabilistic’) knowledge into models in a way that accounts for uncertainty. Based on these two principles, we estimated the amount of uncertainty associated with the location of each stop number (1 to 50) across BBS routes in the central United States by analyzing several existing stop location datasets compiled by other researchers. We then used this information to calculate the resulting uncertainty in forest, grassland, and developed land cover for all BBS routes and years in our study region.
Traditional frequentist modeling approaches assume that covariate values are known without error. However, our Bayesian approach treats land cover covariates in a generalized linear model as parameters to be estimated. In Bayesian models, every estimated parameter is given a ‘prior’ distribution that represents knowledge or beliefs about a quantity prior to modeling. These priors can be broad and vague (‘uninformative’), expressing a lack of knowledge about the quantities, but they can also be specific (‘informative’) when there is some existing information about the values that a parameter is likely to take. We incorporated land cover uncertainty into our models by specifying informative priors for each land cover covariate, which allows the model to consider the full range of land cover values for each stop, and their relative probabilities, rather than assuming a known value that may be misleading. We then use uninformative priors to estimate the real parameters of interest in our model, which quantify the effects of grassland, forest, and develop land on bird communities and account for location stop uncertainty.
Implications for the BBS and beyond
By facilitating fine-scale stop-level analyses, we unlock the tremendous potential of the extensive BBS dataset for answering ecological questions. We’ve made our data and code available so that others can study other questions and species, or expand to other parts of North America.
Many other extensive data sources, including museum records, the Global Biodiversity Information Facility (GBIF), iNaturalist, and eBird also include species records that are associated with considerable location uncertainty. Uncertainty in variables of ecological interest can occur for other reasons, too—for example, variation among climate models or uncertainty in species-level identifications. In each of these cases, a similar method could be used to quantify resulting uncertainty in covariates of interest and incorporate that uncertainty into models using informative priors.